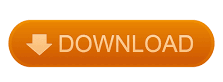
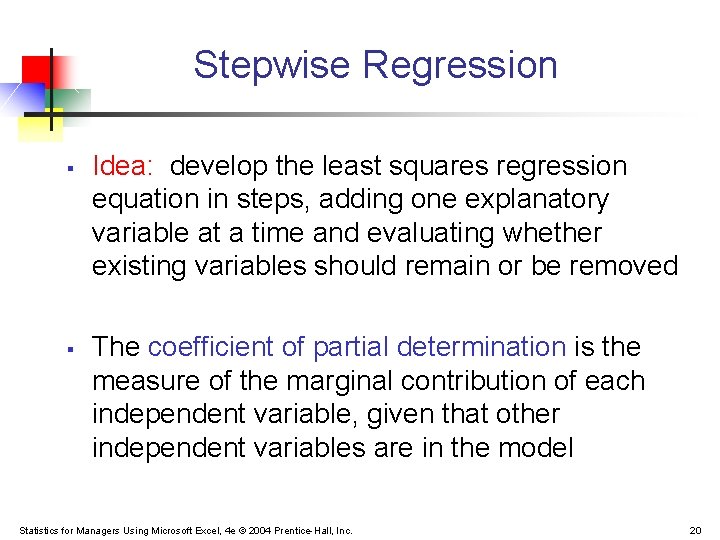
In fact, do not be surprised if your data fails one or more of these assumptions since this is fairly typical when working with real-world data rather than textbook examples, which often only show you how to carry out linear regression when everything goes well.
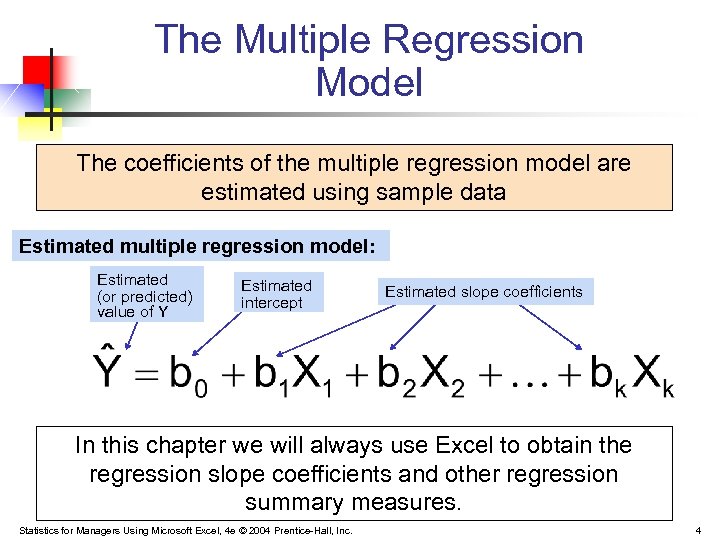
When moving on to assumptions #3, #4, #5, #6, #7 and #8, we suggest testing them in this order because it represents an order where, if a violation to the assumption is not correctable, you will no longer be able to use multiple regression. Note: If you only have categorical independent variables (i.e., no continuous independent variables), it is more common to approach the analysis from the perspective of a two-way ANOVA (for two categorical independent variables) or factorial ANOVA (for three or more categorical independent variables) instead of multiple regression.įortunately, you can check assumptions #3, #4, #5, #6, #7 and #8 using Stata. In this guide, we show you the multiple regression procedure because we have a mix of continuous and categorical independent variables. Examples of categorical variables include gender (e.g., 2 groups: male and female), ethnicity (e.g., 3 groups: Caucasian, African American and Hispanic), physical activity level (e.g., 4 groups: sedentary, low, moderate and high), profession (e.g., 5 groups: surgeon, doctor, nurse, dentist, therapist), and so forth. For examples of continuous variables, see the bullet above. Assumption #2: You have two or more independent variables, which should be measured at the continuous or categorical level.If you are unsure whether your dependent variable is continuous (i.e., measured at the interval or ratio level), see our Types of Variable guide. Examples of such continuous variables include height (measured in feet and inches), temperature (measured in ☌), salary (measured in US dollars), revision time (measured in hours), intelligence (measured using IQ score), reaction time (measured in milliseconds), test performance (measured from 0 to 100), sales (measured in number of transactions per month), and so forth. Assumption #1: Your dependent variable should be measured at the continuous level.However, you should decide whether your study meets these assumptions before moving on. Since assumptions #1 and #2 relate to your choice of variables, they cannot be tested for using Stata. If any of these eight assumptions are not met, you cannot analyze your data using multiple regression because you will not get a valid result. There are eight "assumptions" that underpin multiple regression. However, before we introduce you to this procedure, you need to understand the different assumptions that your data must meet in order for multiple regression to give you a valid result. This "quick start" guide shows you how to carry out multiple regression using Stata, as well as how to interpret and report the results from this test. For example, you might want to know how much of the variation in exam anxiety can be explained by coursework mark, revision time, lecture attendance and IQ score "as a whole", but also the "relative contribution" of each independent variable in explaining the variance. Multiple regression also allows you to determine the overall fit (variance explained) of the model and the relative contribution of each of the independent variables to the total variance explained. If you have a dichotomous dependent variable you can use a binomial logistic regression. Alternately, you could use multiple regression to determine if income can be predicted based on age, gender and educational level (i.e., the dependent variable would be "income", and the three independent variables would be "age", "gender" and "educational level"). For example, you could use multiple regression to determine if exam anxiety can be predicted based on coursework mark, revision time, lecture attendance and IQ score (i.e., the dependent variable would be "exam anxiety", and the four independent variables would be "coursework mark", "revision time", "lecture attendance" and "IQ score"). Multiple regression (an extension of simple linear regression) is used to predict the value of a dependent variable (also known as an outcome variable) based on the value of two or more independent variables (also known as predictor variables). Multiple Regression Analysis using Stata Introduction
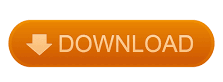